Unveiling the Vulnerabilities of AI-Based Systems in Cybersecurity
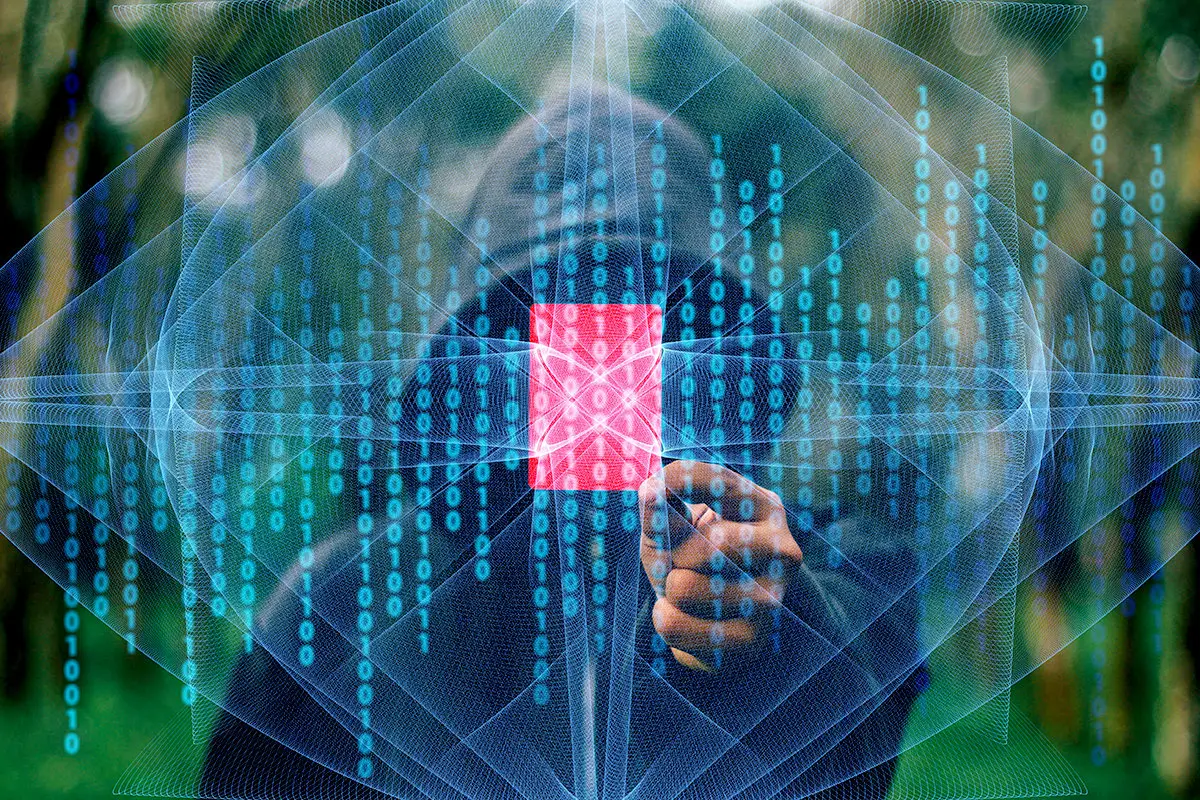
As artificial intelligence (AI) becomes increasingly integrated into our digital landscape, it is crucial to recognize that AI-based systems are not immune to vulnerabilities. In this blog post, we dive deep into the potential weaknesses of AI systems in terms of cybersecurity. By examining specific companies as examples, we shed light on the challenges and risks associated with AI vulnerabilities. Join us as we explore the intricacies of AI-based cybersecurity and how organizations can navigate this rapidly evolving landscape.
One significant vulnerability in AI-based systems is adversarial machine learning, where malicious actors exploit vulnerabilities in AI algorithms to manipulate outputs and deceive defenses. Take DeepInstinct as an example, a company that showcases the potential risks of AI-based cyberattacks. Adversarial AI systems can generate misleading outputs, infiltrate secure systems, and compromise cybersecurity measures. These attacks are designed to exploit the limitations of AI algorithms and deceive AI-based defense mechanisms.
Another vulnerability lies in the security of AI training data. Many AI systems rely on large datasets to train their models, and if these datasets are compromised, it can lead to severe consequences. For instance, consider a company like OpenAI, whose research into AI language models has raised concerns about the dissemination of convincing fake news. If attackers gain unauthorized access to or manipulate the training data, it can result in the generation and spread of misleading or harmful information.
AI models are susceptible to poisoning attacks, where adversaries inject malicious data during the training phase, leading to compromised models. This vulnerability was demonstrated in the case of ChatGPT, an AI language model developed by OpenAI. Attackers can manipulate the training process, introducing biases or malicious behaviors that could go unnoticed until the model is deployed and exploited.
The lack of explainability in AI models poses a significant challenge to cybersecurity. As companies like IBM Watson deploy AI systems for threat detection, it becomes essential to understand how decisions are made. The black-box nature of complex AI algorithms makes it difficult to determine the reasons behind certain actions, leaving potential blind spots in security defenses. Attackers can exploit these blind spots by evading detection or bypassing security measures.
Mitigating the Risks and Strengthening AI-Based Systems (Approx. 600 characters):
While vulnerabilities in AI-based systems exist, organizations can take proactive measures to mitigate risks and strengthen their cybersecurity defenses. Implementing the following practices can help enhance the resilience of AI systems:
- Robust Data Security: Ensuring the security and integrity of training data is crucial. Organizations should implement strong data protection measures, such as encryption and access controls, to safeguard sensitive information from unauthorized access or manipulation.
- Adversarial Training: Incorporating adversarial training techniques during the model development process can enhance the resilience of AI systems against attacks. By exposing models to adversarial examples during training, they can learn to recognize and defend against potential threats.
- Explainable AI: Promoting transparency and explainability in AI models is essential for understanding their decision-making processes. By using interpretable AI techniques, organizations can gain insights into how AI models arrive at their conclusions, improving security and facilitating effective incident response.
- Continuous Monitoring and Testing: Regularly monitoring AI systems for anomalies and conducting rigorous testing helps identify potential vulnerabilities. This includes evaluating the system's response to adversarial attacks, ensuring its ability to detect and respond to emerging threats effectively.
In the rapidly evolving field of AI-based cybersecurity, it is crucial to acknowledge and address the vulnerabilities that AI systems may possess. By understanding the risks associated with adversarial machine learning, data security, model poisoning, and explainability, organizations can take proactive steps to strengthen their AI-based defenses. Embracing robust security measures and continuously monitoring and updating AI systems will play a vital role in mitigating the risks and ensuring a more secure digital landscape.